Bridging Innovation and Compliance in the Age of AI
.jpeg?width=58&height=58&name=1550187579208%20(1).jpeg)
AI is reshaping regulated industries, presenting a critical challenge: maintaining compliance while accelerating innovation.
In high-stakes fields like healthcare, transportation, and manufacturing, the push to adopt AI must align with strict regulatory requirements to ensure safety, quality, and fairness.
This creates a complex dilemma—how to integrate AI and automation while ensuring compliance processes, such as safety protocols and task assessments, align with scalable skills frameworks. Success lies in balancing operational efficiency with the rigorous standards these industries demand.
In the latest Skills Connect episode, I spoke with Gordon Ritchie, Principal Consultant in Skills Architecture and Transformation, about navigating this challenge. We discussed strategies for building frameworks that align tasks, skills, and compliance while embracing AI responsibly.
To bridge innovation and compliance, organizations must focus on three key pillars:
1. Building a Foundation: Clear Task and Skill Definition
In an AI-driven world, precise documentation becomes even more critical. Without clearly defined tasks and skills, organizations cannot effectively validate compliance or leverage AI's potential.
Key Insights:
- Precision drives compliance: Take train driving, for instance. A task like "must not proceed through a red signal" requires explicit documentation that both humans and AI can interpret consistently.
- Technology enables scale: While AI can help analyze and categorize tasks, the foundation must be built on properly harmonized and validated definitions.
"Poorly articulated job descriptions create chaos in regulated industries," notes Gordon. "Organizations need to see beyond individual tasks to create a framework that supports both compliance and innovation."
2. Validating Skills in the AI Era
As AI transforms how work gets done, skill validation must evolve while maintaining its rigor. This is particularly crucial in high-stakes industries where safety and compliance are paramount.
The Triangulation Model:
- Manager Validation: Direct observation and assessment
- HR-Led Validation: Structured certifications and assessments
- Individual Self-Validation: Employee input with external verification
- AI-Enhanced Validation: Platforms like Salesforce or JIRA providing real-time performance data
"The key is creating defensible benchmarks," Gordon emphasizes. "As AI becomes more prevalent, validation methods must withstand increased scrutiny while demonstrating real-world application."
3. Responsible Technology Integration
While AI offers unprecedented opportunities for scaling skills frameworks, its implementation must be thoughtful and controlled, particularly in regulated environments.
Key Considerations:
- AI's Role: Large language models can assist in task analysis but require human validation
- Data Quality: Maintaining accuracy across industries through rigorous harmonization
- Governance: Ensuring fairness and consistency in AI-enhanced compliance systems
"AI must prove its value," Gordon states. "It's not about replacing existing systems but enhancing them through a partnership between human expertise and technological capability."
Looking Ahead: The Future of Compliance and AI
As organizations navigate this transformation, the focus should be on progress over perfection. Success requires:
- A clear vision for integrating AI while maintaining compliance
- Robust frameworks that support both innovation and safety
- Continuous adaptation as technology evolves
The organizations that will thrive are those that can harness AI's potential while strengthening, not compromising, their compliance frameworks. This isn't just about meeting regulatory requirements—it's about building a foundation for sustainable innovation in regulated industries.
The future belongs to organizations that can strike this balance, creating frameworks that are both compliant and capable of driving transformation in the AI era.
Siobhan 💜
- Sign up for our full email newsletter to get weekly resources, exclusive extras, and more delivered straight to your inbox. 📩
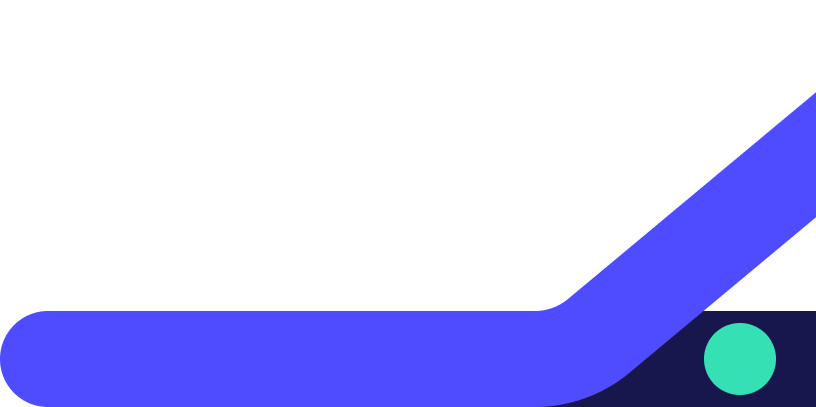